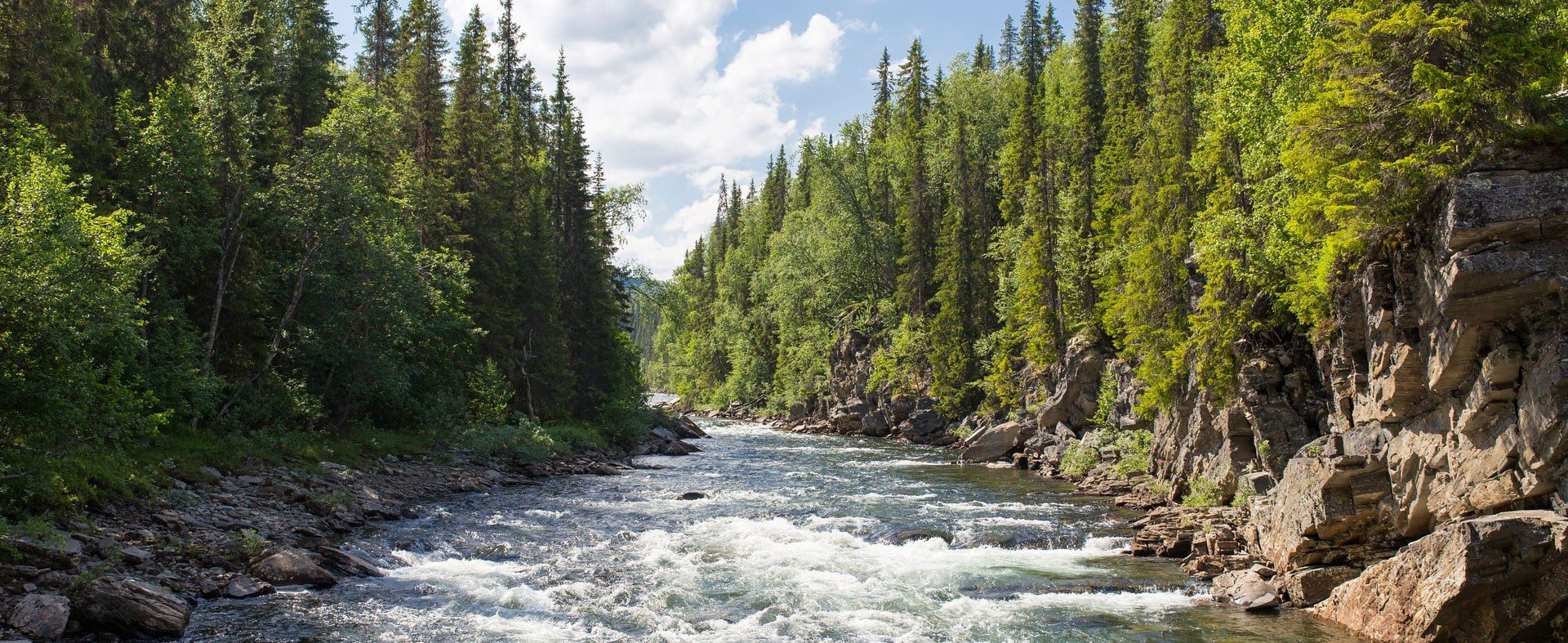
Research Data Management Guide
CUAHSI offers guidance on the creation of data collection and data management plans in order to facilitate the sharing of data and data products.
The 'Research Data and Data Products Guide' provided here is offered for potential general use in defining research products and data sharing workflows. It is intended to be used as an example from which specific data policies, timing, and best practices for data sharing can be defined and adopted for research. This guide has been developed by CUAHSI for general community use, from the iUTAH data policy, with the help of collaborator Jeff Horsburgh.
Expand the panel below to read the plan or download a pdf version.
A standardized set of quality control levels to describe data and data products
This document is adapted from the iUTAH Research Data Policy (Horsburgh and Jones, 2017), which was adopted by a large group of collaborative researchers in Utah working on an NSF-funded research project. It is offered here for potential general use in defining research products and data sharing workflows. This document is intended to be used as an example from which specific data policies, timing, and best practices for data sharing can be defined and adopted for research projects like the Critical Zone Collaborative Network Thematic Cluster projects.
Revised by Clara Cogswell, Shannon Syrstad, Jeff Horsburgh 2/17/2022
Section 1: Purpose
Research data are defined as “the recorded factual material commonly accepted in the scientific community as necessary to validate research findings” by the U.S. Office of Management and Budget and includes the data as well as the metadata that define the content and context of the data (US OMB, 1999). The National Academy of Sciences, National Academy of Engineering, and Institute of Medicine (2009) describe research data:
It includes textual information, numeric information, instrumental readouts, equations, statistics, images (whether fixed or moving), diagrams, and audio recordings. It includes raw data, processed data, published data, and archived data. It includes the data generated by experiments, by models and simulations, and by observations of natural and social phenomena at specific times and locations. It includes data gathered specifically for research as well as information gathered for other purposes that is then used in research. It includes data stored on a wide variety of media including magnetic and optical media.
The National Science Foundation (NSF) requires establishment of policy governing the data collected as part of NSF-funded research efforts. The guidelines in this document are not intended to replace legal and institutional requirements regarding data rights, privacy, and sharing, but are proposed and implemented to meet NSF requirements and should supplement existing institutional data management requirements.
In general, individuals should provide high quality datasets with sufficient metadata for unambiguous use and interpretation. Requirements for data and metadata may be specific to the associated data types, but a standard set of core metadata is required for all datasets to facilitate archival, storage, and cataloging for discovery and retrieval. Individuals should consider the submittal of datasets for sharing and publication as being similar to the submittal of manuscripts to research journals.
Section 2: Data Collection Plans
Most research proposals require a Data Management Plan that defines the types of data to be collected, and other provisions for how data will be managed by a proposed project. Usually limited to two pages, Data Management Plans may lack the level of detail needed to guide successful collection, management, and publication of diverse data products produced by many projects. Additionally, plans for data collection may change and adapt after a project is funded. As an extension of a project’s Data Management Plan, more specific Data Collection Plans can be a useful tool for planning data collection activities, defining the specific data products to be produced, promoting consensus about authorship and contributions to data products, and planning for submission to a reputable repository for sharing and publication. A Data Collection Plan should include the following information:
- A. Identification of the types of data to be created (using the data typology below).
- B. A brief description of the methods that will be used to create the data.
- C. Identification of the data formats that will be used to store the data.
- D. A brief description of the data or data resources to be created (e.g., what are the final
- products that will be shared/published). Data resources may include a listing (or copies)
- of data instruments, summary reports, journal papers or other publications. If data are to
- exist in multiple repositories, then users should share this information and
- provide information approaches for uniformly updating data across repositories.
- E. A description of who will have access to the data during data collection and how the data will be made broadly available after completion and publication, including any
- restrictions on data sharing with explanations.
- F. Information on potential collaborators/co-authors for each data product and anticipated publications.
A template Data Collection Plan is provided as an appendix to this document.
Section 3: Cases Requiring IRB Approval or Review Prior to Data Collection with Human Subjects
Some data related to human subjects are sensitive in nature. Their collection and management will require approval from an Institutional Review Board (IRB). Efforts should be made to formulate the IRB application and design research so that resulting data are available to the largest number of researchers and partners possible. All IRB documentation should be approved and in place before data collection can begin. It is the responsibility of each researcher or data collection team to acquire IRB approval at their respective institution prior to data collection. Collaborative teams should ensure that all IRB requirements across institutions are met.
Data involving human subjects are subject to data sharing obligations with important qualifications. In some cases, data release may require redaction or de-identification by removing direct identifying information (e.g., names, addresses, etc.) as well as indirect identifiers of research participants that could be used to deduce participant identities. In other cases, aggregation of data to a level at which no individual is identifiable may also be required. In cases where de-identification or aggregation is not possible without compromising the integrity of the data set, or where release is expressly prohibited by the approved IRB protocol or other formal agreements, an objective statistical or narrative summary of the data should be developed and released. Details of plans for data availability and anonymization and/or aggregation should be included in the Data Collection Plan (including any related language from IRB applications, and copies of informed consent documents provided to participants). Researchers are encouraged to include clear information about data sharing intentions in the informed consent documents provided to participants.
Section 4: Data management and sharing considerations for qualitative data
Qualitative data may come in a variety of forms, including, but not limited to, audio/video recordings, transcripts, field notes or summaries, photographs, documents (print or electronic), and maps or drawings. Qualitative data begin in raw form and are typically processed iteratively to transform the data into new formats (e.g., audio transcribed into text or text coded into numeric data or thematic interpretations). Processing may occur by hand or assisted by computer software.
Qualitative data should be shared in the form most appropriate for
validating research findings, accounting for restrictions such as those
imposed by an IRB protocol for protection of human subjects. Sharing of
coded data from qualitative information is encouraged, but not required
when it is conducted as part of analytical processes (following OMB Circular A-110).
Section 5: Storage and Archival
Storage and archival are important considerations for data safety and eventual reuse. Secure storage of data during and after data collection is important to guard against data loss, promote collaboration among project teams, and to meet required data protections associated with IRB protocols. Secure storage may be accomplished via services provided through institutions (e.g., Box, Google Drive, etc.) or other providers. Archival of finalized data products in a reputable repository is necessary to ensure that they are findable, accessible, interoperable, and reusable (FAIR) (https://www.go-fair.org/fair-principles/).
HydroShare is an online, collaborative environment for sharing data, models, and code that supports FAIR Data Principles (Horsburgh et al., 2015). HydroShare was developed with support by NSF (NSF OCI1148453, OCI-1148090) in partnership with the Consortium of Universities for the Advancement of Hydrologic Science, Inc. (CUAHSI) and other institutions. HydroShare provides the functionality needed by researchers to share both data and models, use collaborative groups with public and private sharing of resources, and formal publication of research products, including issuing citable digital object identifiers (DOIs). Using a national scale repository such as HydroShare broadens the impact of datasets by making them discoverable by a wider audience. Similarly, streaming datasets published using CUAHSI HIS WaterOneFlow web services that are registered with the CUAHSI Water Data Center, make those data discoverable alongside other nationally published data.
Specific to models, HydroShare provides resources for archiving data and other files related to environmental modeling, including but not limited to, input data, output data, simulation configuration files, model executable files, and data processing workflows. HydroShare Apps operate on specific resource types that allow participants to explore datasets on the web (e.g., GIS raster and vector mapping) before downloading the content locally for further analysis. Furthermore, Jupyter notebooks provide a web environment for in-depth data analysis of HydroShare content using the Python programming language. These tools are an added benefit of archiving data in the HydroShare web platform that can become part of participants’ analysis workflows.
Section 6: Data and Metadata Standards
All data, both raw and derived, regardless of Type (as defined below) should be documented with metadata. Datasets submitted to HydroShare or other repositories should include, at a minimum, the standard metadata elements of the Dublin Core metadata standard (http://dublincore.org/documents/dces/). For static, quantitative datasets (such as experimental data, survey data, organizational data, etc.), metadata should include clear descriptions of variable names, attributes, and value definitions. Submitted metadata should be consistent with the submitted data – i.e., if the submitted data is raw data, variable names, attributes, and value definitions should be for the raw data. Metadata describing finalized or derived data products that are different from the raw data should describe the finalized or derived data.
Time series data, such as streaming sensor data, may be stored using the Consortium of Universities for the Advancement of Hydrologic Science, Inc. (CUAHSI) HydroShare in a Resource or in the CUAHSI Hydrologic Information System (HIS) using an instance of the Observations Data Model (ODM) (Horsburgh et al., 2008).
Geospatial datasets can be shared and published in HydroShare using common geospatial data formats. In some cases, HydroShare will automatically create data services from geospatial datasets using existing Open Geospatial Consortium (OGC) interfaces such as Web Map Services, Web Feature Services, and Web Coverage Services for easy use within Geographic Information Systems (GIS) software. For data accessed via HTTP for download, HydroShare ensures that appropriate metadata descriptions accompany the data download. For all datasets, regardless of restrictions on access, metadata should be publicly available for data discovery purposes.
Model-related datasets, including input datasets for computational models, model results, and the computational models themselves, can also be archived using HydroShare. These products can take many forms including, but not limited to, multidimensional space-time (e.g., NetCDF), timeseries and multi-timeseries (e.g., csv, ODM), geospatial datasets (e.g., GeoTiff, Shapefile), and computational code or scripts. HydroShare includes metadata and functionality specific to these content types. For some content types, HydroShare contains built-in mechanisms for parsing metadata directly from the user provided content to streamline the process of metadata population (e.g., time series and multidimensional space-time). Individuals who are generating model-related datasets are encouraged to use this functionality to share their data.
Section 7: Data Typology
The following is a data typology for categorizing datasets and other products (e.g., model results) so that specific policies related to sharing, access, and timeframes can be appropriately applied. The data types are defined as:
Type A - Primary datasets or research products produced by a research team or at a project level. These include raw and quality controlled sensor data, baseline sampling datasets across facilities and sites, and general datasets collected.
Type B - Datasets or other research products that are created by a specific investigator, student, or coordinated research group to support a particular research question or goal.
Type C - Type A and Type B datasets or products that include personally identifiable information or information about human subjects/participants and are subject to IRB restrictions.
Type D – Datasets or other research products that are subject to licensing, copyright, or use restrictions/agreements from the data source that may prohibit general distribution of the data.
Groups of researchers and/or individual investigators often create derived data or other research products based on shared or published datasets. These derived products typically fall under Type B or C.
Section 8: Timing of Metadata and Dataset Submission and Availability
A metadata record for any datasets falling into the categories given by the data typology above (Type A – D) should be created and submitted for sharing within one month of the onset of data collection. General access to datasets will generally follow a time frame specific to each data type. Suggested timing of data availability as outlined below:
Type A - Where possible, automated data streams should be streamed directly into live databases and made available online in near real time. Quality controlled and derived data products should be published within six months of data collection. All other primary datasets should be published within 3 months of the time they become available (e.g., as soon as results are created).
Type B - Finalized data should be submitted for sharing within one year of the completion of data creation activities. Projects may consider making submission of data collected by students a condition of their successful thesis/dissertation defense. For long running data creation activities (i.e., efforts that last longer than one year), the following should be considered:
- The initial metadata description should be reviewed and updated at least once per year.
- Intermediate data sets should be submitted for archival storage at least every 6 months. These data should not be published or released until the dataset is finalized by the data creator.
- Finalized data should be submitted within one year of collection or by the end of the project, whichever comes first.
Type C - Type C datasets should be subject to time requirements described for Type A and Type B datasets. However, they may require the additional step of anonymization, data transformation, or aggregation with methods described in the Data Collection Plan.
Type D - Type D datasets should be published as soon as possible (within three months) and to the greatest extent allowable by the licensing, copyright, and/or data use agreements under which they were created/procured. Some Type D datasets may be permanently restricted and/or have regulated access limited to identified groups via password or other protections.
Data creators and collectors should have the reasonable expectation for the first rights to analysis and publication. Datasets that do not fall into one of the categories above should be reviewed to determine the appropriate timeframe for publication. Section 9 contains a sample data management workflow that illustrates the order and timing of operations related to sharing and publishing datasets.
Section 9: Example Data Management Workflow
The following is an example data management workflow that is included here to provide guidance to researchers who are creating data. These steps may be carried out by principal investigators, data managers, or delegated researchers on the project.
- Data Collection Plan submitted by the collecting researcher(s) to the data manager. Data manager will review the plan and iterate with the researcher(s) on any feedback. The data manager will provide the plan to the principal investigator or others in the event that it is requested, and otherwise track data collection progress in accordance with the data collection plan.
- Data collection/creation commences.
- Submit metadata to HydroShare: Initial metadata submitted according to the standard metadata format within one month of the onset of data collection/creation.
- Submit data to HydroShare.
- Datasets to be widely accessible should be submitted as soon as they are available.
- Datasets created by graduate student research may be considered a condition of their successful thesis/dissertation defense.
- Datasets created by others should be submitted within one year of the completion of data collection/creation.
- Data subsets/updates for long running datasets should be submitted at least every 6 months.
- Datasets should be shared publicly within one month of successful submission.
- Authors should formally publish their data resources via HydroShare to obtain digital object identifiers (DOIs) and promote citation of their published products.
Section 10: Steps for Sharing/Publishing Data and Models
The steps required for sharing data products vary by repository. As an example, the following are the steps that should be completed by data managers or investigators to upload, share, and publish datasets in the HydroShare and EarthChem repositories:
For HydroShare:
- Create a HydroShare Account at https://www.hydroshare.org.
- Create a HydroShare resource. At minimum, this requires a title, an abstract, and at least one keyword.
- Add content files to the resource. For the resource to be shared or discovered, at least one file must be added. HydroShare will handle files of any format. For each resource, multiple files can be loaded, and files may be organized into folders. Files may include data results, detailed metadata, documentation of data collection methods, model results, or model input files.
- Add relevant metadata. Add authors and contributors to the dataset. Add funding agency credits.
- Give ownership privileges to the Data Manager. Note that ownership does not imply authorship.
- Share the resource with relevant HydroShare Groups.
- Set
Data Sharing Level: This should be done according to the resource’s
status and agreed upon level of availability listed in the Data
Collection Plan you submitted.
- Private: Only HydroShare users with specific permission can discover and access the resource.
- Discoverable: Anyone can discover the resource, but only HydroShare users with permission can access the content files.
- Public: Anyone can discover the resource and access the resource’s content files.
- Shareable: Anyone you have given access to the resource can give other users access at the same level.
- Formally Publish Your resource: Formally publishing your resource assigns a digital object identifier that can be cited, makes the content files, resource title, and authors immutable (so that the citation will not change), and should be viewed similar to publishing a research paper. This is a FINAL step and should ONLY be done when you are SURE that the content files, title, and authorship of your resource are complete. Metadata such as the abstract, keywords, and related resources can still be edited for published resources.
For EarthChem:
- Log into Earthchem using OrcID or GeoPass
- Review the checklist, submission guidelines, and FAQ, as well as the data templates linked at the top of the data submission form.
- Complete the data submission form and indicate a data release date. Reset the page if needed with the yellow reset button, otherwise save progress, or submit data.
REFERENCES
DataONE Project Team. 2011. DataONE structure and potential partnership as a member node. Accessed: September 14, 2011. Available from: http://www.dataone.org/content/dataonestructure-and-potential-member-node.
de la Beaujardiere , J. (editor) 2006. Open GIS web page map server implementation specification, OGC implementation specification OGC 06-042, version 1.3.0, http://portal.opengeospatial.o..., pp.
Horsburgh, J.S., D.G. Tarboton, D.R. Maidment, and I. Zaslavsky. 2008. A Relational Model for Environmental and Water Resources Data. Water Resour. Res. 44:W05406.
Horsburgh, J.S., D.G. Tarboton, M. Piasecki, D.R. Maidment, I. Zaslavsky, D. Valentine, and T.Whitenack. 2009. An integrated system for publishing environmental observations data. Environmental Modelling & Software 24(8):879-888. Horsburgh, J.S., D.G. Tarboton, D.R. Maidment, and I. Zaslavsky. 2010a. Components of an integrated environmental observatory information system. Computers & Geosciences 37(2):207-218.
Horsburgh, J.S., D.G. Tarboton, K.A.T. Schreuders, D.R. Maidment, I. Zaslavsky, and D. Valentine. 2010b. Hydroserver: A Platform for Publishing Space-Time Hydrologic Datasets. in 2010 AWRA Spring Specialty Conference Geographic Information Systems (GIS) and Water Resources VI. Orlando Florida, American Water Resources Association, Middleburg, Virginia, TPS-10-1.
Horsburgh, J.S., M.M. Morsy, A.M. Castronova, J.L. Goodall, T. Gan, H. Yi, M.J. Stealey, D.G. Tarboton. 2015. HydroShare: Sharing Diverse Environmental Data Types and Models as Social Objects with Application to the Hydrology Domain. Journal of the American Water Resources Association. 52(4). DOI: 10.1111/1752-1688.12363
National Academy of Sciences, National Academy of Engineering, and Institute of Medicine. Committee on Ensuring the Utility and Integrity of Research Data in a Digital Age; Committee on Science, Engineering, and Public Policy (COSEPUP); Policy and Global Affairs (PGA); Institute of Medicine (IOM); National Academy of Sciences. 2009. Ensuring the Integrity, Accessibility, and Stewardship of Research Data in the Digital Age. National Academies Press. Washington DC. ttp://www.nap.edu/catalog.php?record_id=12615
United States Office of Management and Budget (US OMB). 1999. OMB Circular A-110, "Uniform Administrative Requirements for Grants and Agreements with Institutions of Higher Education, Hospitals, and Other Non-Profit Organizations", http://www.whitehouse.gov/omb/circulars_a110#36
Vretanos, P.A. (editor) 2010. Open GIS web feature service 2.0 interface standard. OGC implementation standard OGC 09-025r1 and IOS/DIS 19142, version 2.0.0, http://portal.opengeospatial.o..., pp. Whiteside, A. and J.D. Evans. 2008. Web coverage service (WCS) implementation standard. OGC implementation standard OGC 07-067r5, version 1.1.2, http://portal.opengeospatial.o..., pp.
Zaslavsky, I., D. Valentine, and T. Whiteaker. 2007. CUAHSI WaterML,
Open Geospatial Consortium Discussion Paper OGC 07-041r1. Accessed.
Version 0.3.0: [Available from:
http://portal.opengeospatial.org/files/?artifact_id=21743.]
Appendix 1: Sample Data Collection Plan
Effort Name:
Provide a tentative name for the data collection effort - e.g., “Data related to nitrogen cycling from the atmosphere to soils to streams” or “Continuous monitoring data for the Logan River Observatory”
Collaborators:
Provide a list of the names for those who will be collaborating on data collection and creation of data products. If the same collaborators will not be authors for all of the products defined in the table below, consider listing authorship for each product here.
Brief Summary :
Provide a brief summary (1-2 paragraphs) of the data collection effort, including a description of the data collection methods, timing and location of data collection, the parties responsible, etc.
Individual(s) Responsible for Metadata Completion:
Provide the names of individuals who will be responsible for generating the metadata describint the data products produced.
Datasets Expected to be Generated:
In this table, identify and describe the specific data products that will be produced. Data “products” should be thought of as individual datasets or other aggregations of data that will be shared together and will receive a dataset citation. Each product should have a separate row in the table. The following list describes what should be included within each column in the table:
- Dataset Title: Provide a descriptive title for the dataset.
- Data Type: Indicate the type of data using the data typology defined in this document and following the table.
- Method of Creation: Include description of data collection and analytical methods. Include sampling methods where appropriate.
- Resulting Data Format: Describe the format of the resulting data files.
- Data Storage: Describe where the data will be stored during data collection and prior to final publication.
- Final Data Product: Describe the artifacts that will be part of the final data product.
- Time Frame: Describe the time frame for data collection and sharing.
- Access During Collection: Describe who will have access to the data during data collection. Indicate access level and point of contact for access.
- Access After Completion: Describe who will have access to the data after it is complete.
- Anonymization for IRB: Describe whether anonymization of the data is required and provide a brief description of methods.
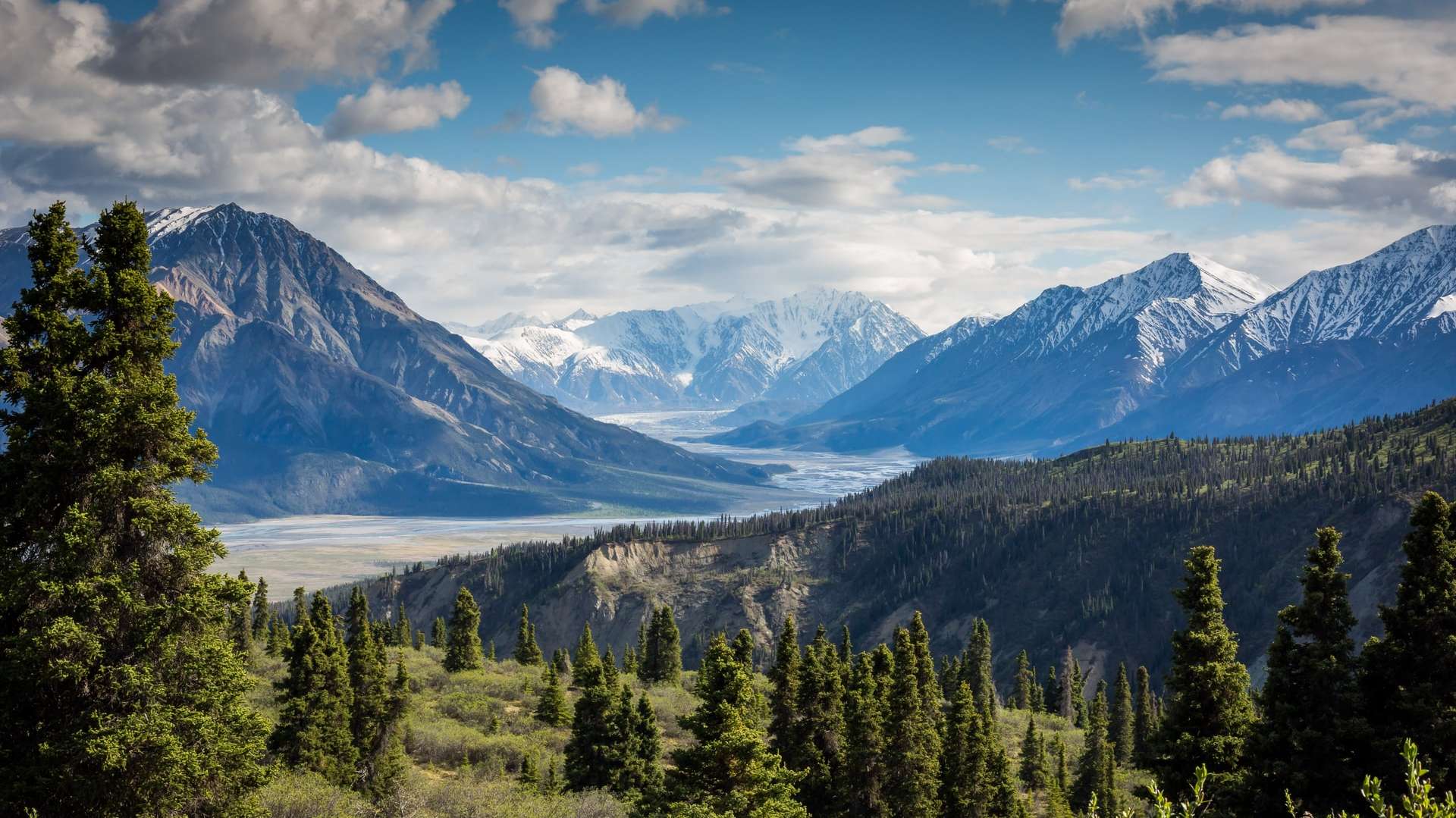